Scale AI Infrastructure: Master Capacity Planning, Maximize Performance, Minimize Costs
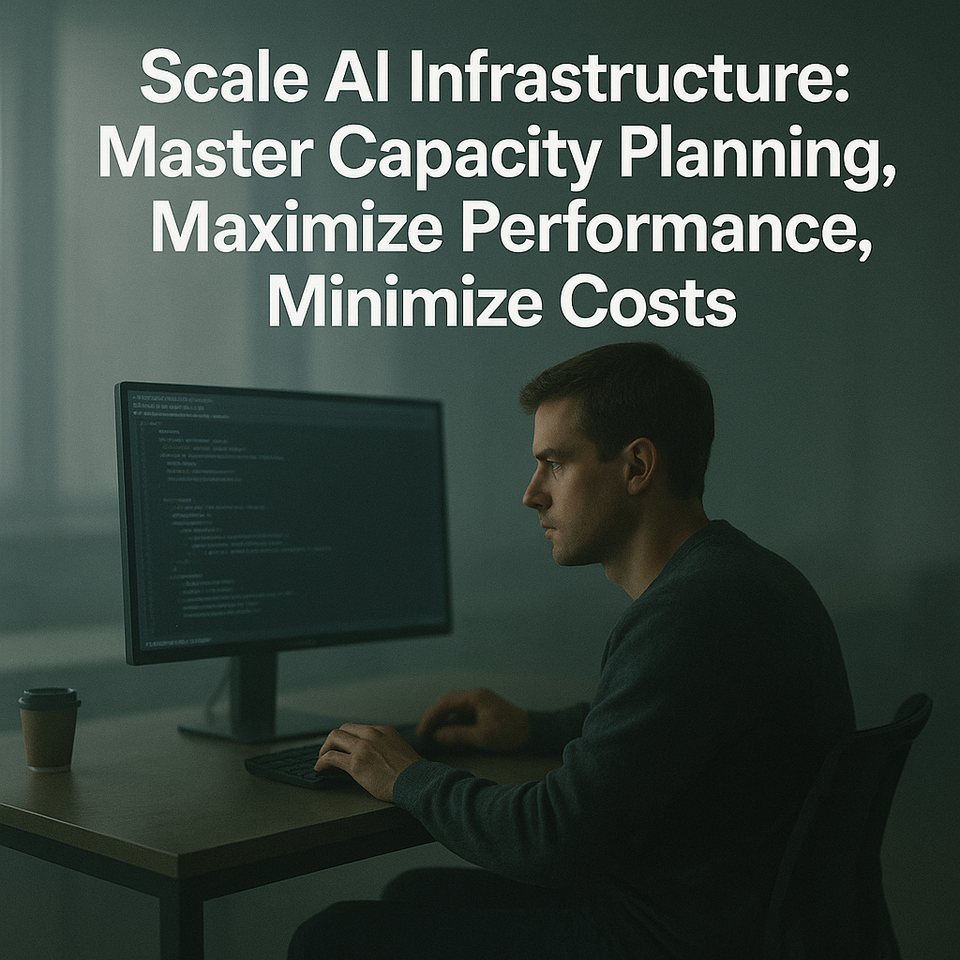
Don't Let AI Capacity Constraints Cripple Your Innovation
Imagine this: your cutting-edge AI model is finally ready for deployment, promising to revolutionize your business. But as user traffic surges, performance grinds to a halt, leaving you with frustrated customers and a stalled project. This nightmare scenario is all too common, highlighting the critical need for robust capacity planning in the world of AI.
The AI Capacity Planning Conundrum: A Growing Challenge
AI workloads are notoriously resource-intensive, demanding significant computational power, memory, and storage. Unlike traditional applications with predictable usage patterns, AI models often exhibit highly variable resource consumption depending on factors like data size, model complexity, and inference load. This makes accurate capacity planning a complex and ongoing challenge.
Failing to adequately plan for AI capacity can lead to a range of problems, including:
- Performance bottlenecks: Slow response times, degraded user experience, and missed SLAs.
- Increased costs: Over-provisioning resources to compensate for uncertainty, leading to wasted investment.
- Delayed deployments: Inability to scale AI models to meet demand, hindering innovation and time-to-market.
- System instability: Overloading infrastructure, resulting in crashes and downtime.
Addressing these challenges requires a strategic approach that considers the unique characteristics of AI workloads and leverages the right tools and techniques.
Unlocking Peak Performance: Strategies for Effective AI Capacity Planning
Effective AI capacity planning involves a holistic approach that encompasses several key areas:
- Workload Characterization: Understanding the resource requirements of your AI models is paramount. This includes analyzing CPU utilization, memory consumption, disk I/O, network bandwidth, and GPU usage (if applicable) under varying load conditions. Tools like profilers and monitoring dashboards can provide valuable insights into workload behavior. This data will form the foundation of your capacity planning model.
- Resource Forecasting: Based on workload characterization, you need to forecast future resource demands. This involves considering factors like anticipated user growth, model updates, data volume increases, and seasonal variations. Statistical forecasting techniques, machine learning models, and expert judgment can be used to predict future resource needs.
- Infrastructure Optimization: Optimize your infrastructure to efficiently support AI workloads. This includes selecting the right hardware (e.g., CPUs, GPUs, memory, storage), configuring the operating system and virtualization environment, and implementing efficient data storage and retrieval mechanisms. Consider using cloud-based services to leverage their scalability and flexibility.
- Capacity Modeling and Simulation: Develop a capacity model that simulates the performance of your AI models under different load scenarios. This allows you to identify potential bottlenecks and optimize resource allocation. Simulation tools can help you evaluate different infrastructure configurations and capacity planning strategies.
- Monitoring and Alerting: Implement comprehensive monitoring and alerting systems to track resource utilization and identify potential capacity issues in real-time. Set up alerts for critical metrics like CPU utilization, memory consumption, and disk I/O to proactively address problems before they impact performance.
- Dynamic Scaling: Implement dynamic scaling mechanisms to automatically adjust resource allocation based on real-time demand. This allows you to optimize resource utilization and avoid over-provisioning. Cloud-based services provide built-in dynamic scaling capabilities.
- Cost Optimization: Balance performance requirements with cost considerations. Explore options like using spot instances, reserved instances, and autoscaling to optimize cloud spending. Implement cost monitoring and reporting to track cloud costs and identify areas for improvement.
- Version Control and Management: Managing AI models and their associated configurations is crucial. Tools like GitScrum allow for efficient version control, ensuring that you can easily revert to previous versions if needed and track changes over time. This is particularly important when optimizing models for performance, as changes can sometimes introduce unexpected issues. GitScrum's project management capabilities also facilitate collaboration between data scientists, engineers, and operations teams, ensuring that everyone is aligned on capacity planning goals.
- Collaboration and Communication: Effective capacity planning requires close collaboration between different teams, including data scientists, engineers, and operations personnel. Establish clear communication channels and processes to ensure that everyone is informed about capacity planning goals, progress, and challenges. GitScrum provides a centralized platform for communication and collaboration, facilitating seamless coordination between teams.
By implementing these strategies, you can significantly improve the performance and efficiency of your AI infrastructure, reduce costs, and accelerate innovation.
Leveraging GitScrum for Streamlined AI Project Management and Capacity Planning
Managing AI projects effectively requires a robust project management tool. GitScrum offers a comprehensive suite of features designed to streamline AI project workflows, from initial planning to deployment and ongoing maintenance. Specifically, GitScrum can significantly aid in capacity planning by:
- Centralized Task Management: GitScrum provides a centralized platform for managing all tasks related to AI model development, deployment, and capacity planning. This ensures that everyone is aligned on priorities and deadlines.
- Agile Workflow Support: GitScrum supports agile methodologies, allowing you to iterate quickly and adapt to changing requirements. This is particularly important in the fast-paced world of AI, where models and data are constantly evolving.
- Progress Tracking and Reporting: GitScrum provides real-time progress tracking and reporting capabilities, allowing you to monitor the status of AI projects and identify potential bottlenecks. This helps you proactively address capacity issues and ensure that projects stay on track.
- Resource Allocation and Management: GitScrum facilitates efficient resource allocation and management, allowing you to assign tasks to specific team members and track their progress. This helps you optimize resource utilization and avoid over-allocation.
- Communication and Collaboration: GitScrum provides a centralized platform for communication and collaboration, facilitating seamless coordination between data scientists, engineers, and operations teams. This ensures that everyone is informed about capacity planning goals, progress, and challenges.
- Documentation and Knowledge Sharing: GitScrum allows you to create and store documentation related to AI models, data, and infrastructure. This helps ensure that knowledge is shared across the team and that everyone has access to the information they need.
- Integration with Other Tools: GitScrum integrates with other popular development tools, such as Git, Jenkins, and Slack, streamlining the AI development workflow.
- Improved Visibility: GitScrum enhances visibility into ongoing AI projects, allowing stakeholders to track progress and identify potential roadblocks early on. This increased transparency fosters better communication and collaboration, leading to more efficient capacity planning.
By leveraging GitScrum, you can streamline your AI project management processes and improve the accuracy and effectiveness of your capacity planning efforts. This will help you optimize resource utilization, reduce costs, and accelerate the deployment of your AI models.
Take Control of Your AI Future: Implement a Proactive Capacity Plan
Don't let capacity constraints hold back your AI innovation. By implementing a proactive capacity planning strategy and leveraging the right tools, you can ensure that your AI models have the resources they need to perform optimally. Remember to continuously monitor your infrastructure, forecast future resource demands, and optimize your resource allocation.
By embracing a data-driven approach to capacity planning, you can unlock the full potential of your AI investments and drive significant business value. Explore how GitScrum can help you manage your AI projects more effectively and streamline your capacity planning efforts.
In conclusion, mastering capacity planning for AI workloads is crucial for maximizing performance, minimizing costs, and driving innovation. By understanding workload characteristics, forecasting resource demands, optimizing infrastructure, and leveraging tools like GitScrum for project management and collaboration, you can ensure that your AI initiatives are successful. Don't wait until performance bottlenecks cripple your projects. Start planning your AI capacity today! Learn more about how GitScrum can help you achieve your AI goals. Explore GitScrum now.