AI-Powered Bug Prevention: Crafting Robust Software Through Automated Defect Mitigation
Explore AI-powered bug prevention and automated defect mitigation for robust software. Learn how AI enhances quality and streamlines development. Check out GitScrum!
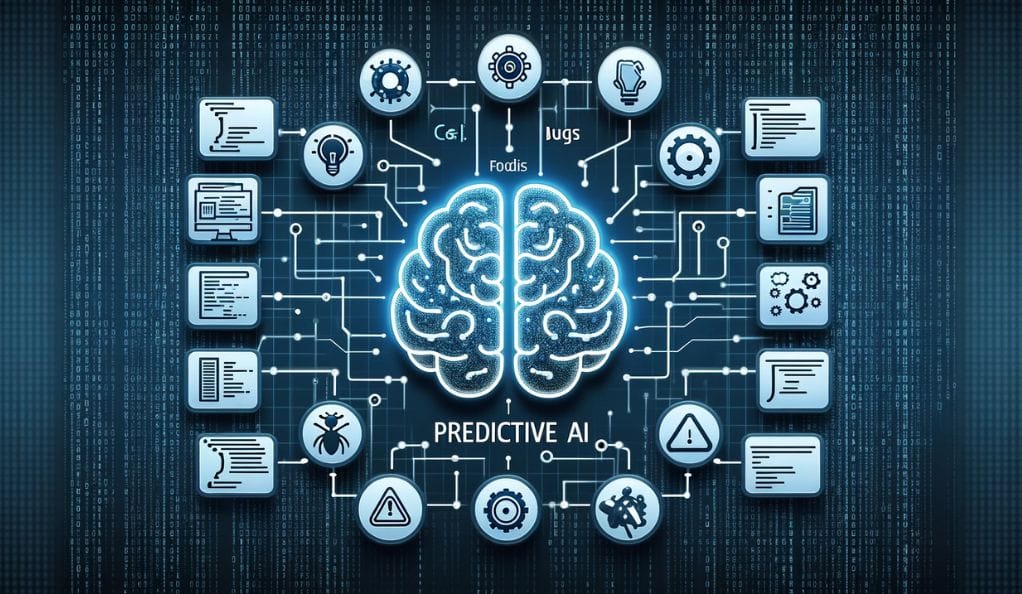
AI-Powered Bug Prevention: Crafting Robust Software Through Automated Defect Mitigation
The escalating complexity of modern software development demands innovative approaches to ensure quality and reliability. AI-powered bug prevention is rapidly emerging as a critical tool, offering proactive solutions to identify and mitigate potential defects before they impact the end-user. Traditional testing methods, while valuable, often struggle to keep pace with the speed and scale of contemporary development cycles. AI offers a transformative approach by automating many aspects of the testing and defect detection process, resulting in more robust and reliable software. This is crucial for maintaining user trust and minimizing the costly consequences of software failures.
Shifting Left: Proactive Defect Detection with AI
The concept of "shifting left" in software development emphasizes early defect detection. Integrating AI-driven tools into the development lifecycle can significantly enhance this proactive approach. By analyzing code during the writing phase, AI algorithms can identify potential vulnerabilities, coding errors, and design flaws that might otherwise slip through traditional review processes. This early intervention reduces the cost and complexity of fixing bugs later in the development cycle.
Static analysis tools, augmented with AI, can learn from vast codebases and identify patterns associated with known bugs. These tools can flag suspicious code sections, suggesting potential improvements or alternative implementations. Furthermore, AI can automate the process of generating unit tests, ensuring that code is thoroughly tested from the outset. For example, an AI-powered tool might analyze a newly written function and automatically generate a suite of tests that cover various input scenarios and edge cases. This significantly reduces the manual effort required for unit testing and improves code coverage.
Consider the impact of using machine learning models to predict potential bugs based on code complexity metrics. Studies have shown a correlation between code complexity and the likelihood of defects. AI can analyze code for cyclomatic complexity, nesting depth, and other complexity measures, identifying modules that are more prone to errors. Developers can then focus their attention on these high-risk areas, refactoring code or adding additional tests to mitigate potential issues. AI-driven code analysis is a powerful technique for enhancing software quality and reducing the incidence of bugs.
Automated Code Review and Vulnerability Scanning
Traditional code review processes can be time-consuming and subjective. AI can automate many aspects of code review, providing objective and consistent feedback to developers. AI-powered code review tools can analyze code for adherence to coding standards, potential security vulnerabilities, and performance bottlenecks. These tools can identify common coding errors, such as null pointer exceptions, buffer overflows, and SQL injection vulnerabilities, before they are committed to the codebase.
Furthermore, AI can learn from past code reviews and identify patterns of defects that are specific to a particular project or team. This allows the AI to provide more targeted and relevant feedback, helping developers to avoid making the same mistakes repeatedly. Automated vulnerability scanning is another critical application of AI in software development. AI-powered scanners can automatically identify known vulnerabilities in third-party libraries and frameworks, alerting developers to potential security risks. This is particularly important in today's development landscape, where software often relies on a complex ecosystem of external dependencies.
- Static Analysis: Identifies potential issues without executing the code.
- Dynamic Analysis: Analyzes code behavior during runtime.
- Fuzzing: Generates random inputs to uncover unexpected behavior and vulnerabilities.
- Machine Learning-Based Prediction: Predicts potential defects based on code characteristics.
Harnessing AI for Dynamic Testing and Defect Prediction
While static analysis focuses on code structure and syntax, dynamic testing involves executing the code and observing its behavior. AI-powered dynamic testing can automate many aspects of the testing process, including test case generation, test execution, and defect reporting. AI algorithms can analyze code coverage data to identify areas that are not adequately tested and automatically generate new test cases to improve coverage. This ensures that all parts of the code are thoroughly tested, reducing the likelihood of undiscovered bugs.
Furthermore, AI can be used to optimize test execution, prioritizing tests that are more likely to uncover defects. By analyzing historical test results and code changes, AI can identify tests that have a higher probability of failure and prioritize them for execution. This reduces the overall testing time and allows developers to focus on the areas that are most likely to contain bugs. One powerful technique is using AI to predict defects based on code changes. Machine learning models can be trained on historical code changes and bug reports to identify patterns that are indicative of potential defects. These models can then be used to predict which code changes are most likely to introduce bugs, allowing developers to focus their testing efforts on these high-risk areas.
Consider the application of AI in automating UI testing. Traditional UI testing can be tedious and time-consuming, requiring manual interaction with the application. AI-powered UI testing tools can automatically generate test scripts that simulate user interactions and verify that the application behaves as expected. These tools can also automatically detect visual regressions, such as changes in layout or font size, that might indicate a bug. By automating UI testing, developers can significantly reduce the time and effort required to ensure the quality of their applications. If your team uses agile methodologies, GitScrum can help streamline your workflow and integrate AI-driven testing results for enhanced project visibility.
Augmenting Agile Workflows with AI-Driven Insights
Agile development methodologies emphasize iterative development and continuous feedback. Integrating AI-driven tools into agile workflows can further enhance the speed and quality of software development. For instance, AI can automate the process of triaging bug reports, prioritizing them based on severity and impact. This ensures that the most critical bugs are addressed first, minimizing the risk of disrupting the development process. GitScrum helps teams visualize their progress and manage tasks within an agile framework.
AI can also be used to analyze code churn and identify areas that are undergoing frequent changes. These areas are often more prone to bugs, so developers can focus their testing efforts on them. Furthermore, AI can provide insights into team performance, identifying areas where developers are struggling or where the development process can be improved. For example, AI can analyze code review data to identify developers who are consistently making the same mistakes, providing them with targeted training to improve their skills. By providing data-driven insights into team performance, AI can help to optimize the development process and improve the overall quality of the software. Using GitScrum, teams can track progress, manage sprints, and leverage AI-driven insights for continuous improvement.
Here is how an AI-driven system can enhance the agile workflow:
- Prioritize bug reports based on predicted severity and impact.
- Automate test case generation for new features.
- Identify code areas with high churn rates for focused testing.
- Provide insights into team performance and identify training needs.
- Suggest optimal sprint planning based on historical data and team velocity.
By leveraging these AI-driven capabilities, agile teams can significantly improve their efficiency and deliver higher-quality software faster. This integration also leads to better resource allocation and more informed decision-making throughout the development lifecycle.
Embrace AI for Superior Software Quality
The future of software development is undoubtedly intertwined with artificial intelligence. From proactive defect detection to automated testing and insightful team performance analysis, AI offers a powerful suite of tools for enhancing software quality and streamlining the development process. By embracing AI-powered bug prevention, organizations can build more robust, reliable, and secure software, ultimately leading to greater customer satisfaction and business success. Explore how GitScrum can help your team manage projects and integrate AI-driven insights for a more efficient workflow.
In conclusion, AI-powered bug prevention represents a paradigm shift in software development. By automating key aspects of the testing and defect detection process, AI enables developers to build higher-quality software faster and more efficiently. Embracing AI in your development workflow is no longer a luxury but a necessity for staying competitive in today's rapidly evolving technology landscape. Ready to elevate your software quality? Explore GitScrum and discover how it can integrate with AI tools to streamline your development process and reduce bugs.