AI Agile Forecasts Sprint Success – Optimize Project Delivery Through Predictive Insights
Predict sprint success with AI! Optimize resource allocation and improve project delivery through data-driven insights. Discover AI-powered agile.
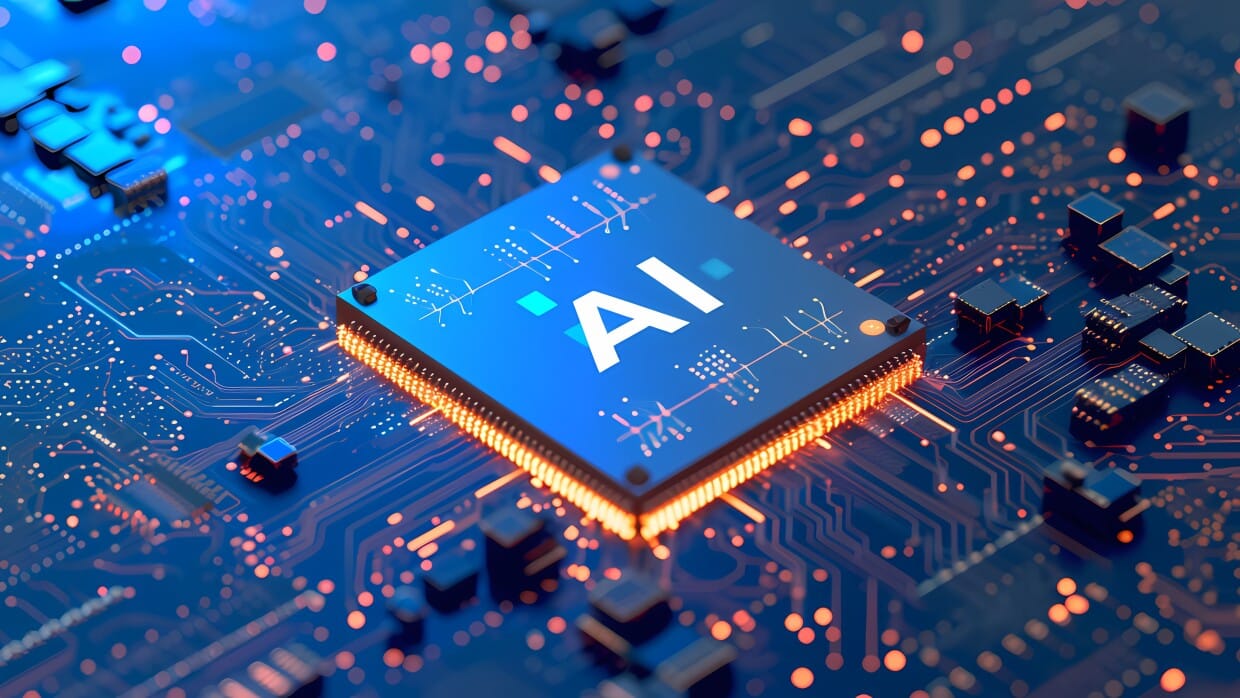
AI Agile Forecasts Sprint Success – Optimize Project Delivery Through Predictive Insights
The relentless pressure to deliver software faster, with higher quality, and within budget is a constant challenge for agile development teams. Traditional sprint planning often relies on gut feeling and historical data, leading to inaccurate estimations, scope creep, and ultimately, sprint failures. Many teams struggle with predicting sprint success, and this unpredictability can result in missed deadlines, frustrated stakeholders, and decreased team morale.
Unveiling the Hidden Bottlenecks in Sprint Execution
Without accurate sprint forecasting, teams are essentially flying blind. Inefficient resource allocation, unforeseen dependencies, and underestimated task complexities frequently derail even the most meticulously planned sprints. This lack of visibility into potential roadblocks leads to reactive firefighting, context switching, and a general sense of chaos. Consider the impact: a recent study showed that nearly 40% of software projects fail to meet their initial deadlines, costing companies millions in lost revenue and wasted resources. Furthermore, constant sprint overruns erode trust between development teams and stakeholders, creating a cycle of negativity and hindering future project success.
The reliance on manual data analysis and subjective estimations further exacerbates the problem. Historical velocity, while useful, often fails to account for the nuances of each individual sprint, such as team composition changes, new technologies, or evolving requirements. This leads to inaccurate forecasts and a false sense of security. The result is often a frantic scramble to catch up towards the end of the sprint, leading to rushed code, increased bug counts, and ultimately, a compromised product.
Technical debt accumulates rapidly when teams are forced to cut corners to meet unrealistic deadlines. This debt, in turn, slows down future development efforts and increases the risk of critical system failures. The cost of fixing bugs in production is significantly higher than fixing them during the development phase, highlighting the importance of proactive sprint management and accurate AI-powered agile predictions.
Quantifying the Impact of Inaccurate Sprint Predictions
Let's break down the tangible costs associated with poor sprint predictability:
- Increased Development Costs: Rework, bug fixes, and extended development cycles significantly inflate project budgets.
- Missed Market Opportunities: Delayed product releases allow competitors to gain a foothold in the market.
- Reduced Team Morale: Constant pressure to meet unrealistic deadlines leads to burnout and decreased productivity.
- Compromised Product Quality: Rushed code and inadequate testing result in a subpar user experience.
- Damaged Stakeholder Relationships: Missed deadlines erode trust and create friction between development teams and stakeholders.
These factors combined create a significant drag on organizational performance, hindering innovation and impacting the bottom line. A shift towards data-driven agile sprint planning is crucial for mitigating these risks and maximizing the return on investment in software development.
Harnessing AI for Data-Driven Sprint Forecasting and Improved Agile Execution
Imagine a world where sprint planning is based on data-driven insights, where potential roadblocks are identified proactively, and where teams can confidently predict their sprint outcomes. This is the promise of AI in agile project management. By leveraging machine learning algorithms, development teams can analyze vast amounts of historical data, identify patterns, and generate accurate sprint forecasts. This allows for more realistic sprint planning, better resource allocation, and ultimately, increased sprint success rates.
AI-powered agile forecasting goes beyond simply analyzing historical velocity. It takes into account a wide range of factors, including team member skills, task dependencies, code complexity, and even external factors such as holidays and company events. This holistic approach provides a much more accurate and nuanced view of the sprint landscape, allowing teams to make informed decisions and proactively mitigate potential risks.
Tools like GitScrum facilitate enhanced task management, project organization, and team collaboration, contributing to a more predictable and successful sprint outcome. By leveraging GitScrum's features, teams can better visualize their workflow, track progress, and identify potential bottlenecks early on.
Implementing AI-Driven Agile with GitScrum
Here's how development teams can leverage GitScrum and other tools to implement AI-driven agile:
- Data Collection and Preparation: Gather historical sprint data, including task estimates, actual completion times, bug counts, and team member performance metrics. Ensure data is clean, consistent, and properly formatted.
- AI Model Training: Train a machine learning model using the historical data. Experiment with different algorithms, such as regression models, time series analysis, and neural networks, to find the best fit for your specific needs.
- Sprint Forecasting: Use the trained AI model to generate sprint forecasts based on the current sprint backlog, team composition, and other relevant factors.
- Real-time Monitoring and Adjustment: Continuously monitor sprint progress and compare it to the AI-generated forecasts. Identify any deviations and make necessary adjustments to the sprint plan.
- Feedback and Iteration: Collect feedback from the development team and stakeholders on the accuracy of the AI forecasts. Use this feedback to refine the AI model and improve its predictive capabilities. GitScrum can help facilitate this feedback loop through its collaborative features.
Benefits of AI-Enhanced Agile Project Management
The advantages of incorporating artificial intelligence in agile are numerous and far-reaching:
- Improved Sprint Predictability: Accurate sprint forecasts enable teams to plan more effectively and reduce the risk of missed deadlines.
- Enhanced Resource Allocation: AI-powered insights help teams allocate resources more efficiently, ensuring that the right people are working on the right tasks at the right time.
- Proactive Risk Mitigation: Early identification of potential roadblocks allows teams to proactively address issues before they impact sprint progress.
- Increased Team Productivity: Streamlined workflows and reduced context switching lead to increased team productivity and higher-quality code. GitScrum's task management features can further boost productivity.
- Improved Stakeholder Satisfaction: Consistent sprint delivery and transparent communication build trust and strengthen relationships with stakeholders.
By embracing AI-driven agile, development teams can transform their project management practices and achieve significant improvements in sprint success rates, product quality, and overall organizational performance. Furthermore, tools like GitScrum provide the necessary infrastructure for implementing and managing AI-enhanced agile workflows.
In conclusion, AI in agile development offers a powerful solution for overcoming the challenges of traditional sprint planning. By leveraging machine learning algorithms and tools like GitScrum, teams can gain valuable insights into their development processes, improve sprint predictability, and ultimately deliver higher-quality software faster. Ready to transform your agile practices? Visit GitScrum to learn more about how our platform can help you implement AI-driven sprint forecasting and achieve greater project success.