AI Agents Amplify Project Bottlenecks?
Avoid project bottlenecks! Learn how AI Agents empower teams via intelligent automation and strategic project management with tools like GitScrum.
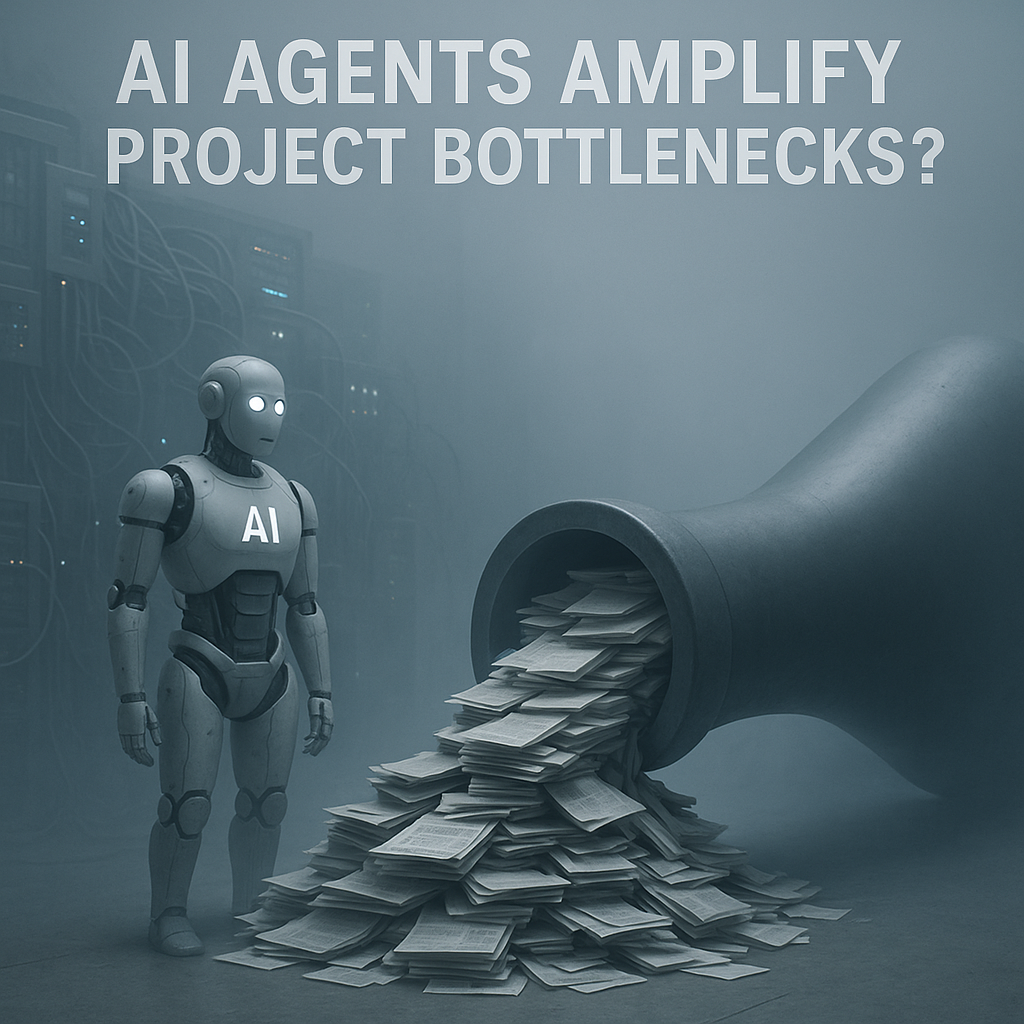
Empowering Teams with Intelligent Automation
The relentless pursuit of efficiency in software development and data science projects often encounters a significant hurdle: project bottlenecks. These bottlenecks, frequently stemming from repetitive tasks, communication breakdowns, and inefficient resource allocation, can severely impact project timelines, budgets, and overall team morale. The integration of AI Agents into project workflows promises a transformative shift, but without proper planning and execution, these agents can inadvertently exacerbate existing problems or introduce new complexities.
Workflow Gridlock: The Stranglehold on Project Velocity
Many software development teams struggle with a complex web of interconnected tasks, dependencies, and communication channels. Identifying the critical path and managing dependencies becomes a constant battle. The introduction of AI Agents, while intended to automate certain processes, can actually contribute to workflow gridlock if not carefully integrated. For instance, an AI Agent designed to generate initial code scaffolding might inadvertently create incompatible code snippets or introduce vulnerabilities if not properly aligned with the team's coding standards and security protocols.
Furthermore, the lack of clear ownership and responsibility for AI Agent outputs can lead to confusion and rework. If developers are unsure who is accountable for verifying and validating the agent's work, errors can propagate through the codebase, leading to costly debugging efforts. Data science projects face similar challenges. An AI Agent tasked with data preprocessing might introduce biases or inaccuracies if not thoroughly audited and validated by domain experts. The potential for these issues to compound over time creates a significant risk to project success. The problem of inefficient task management is further compounded when teams lack robust tools for visualizing workflows and tracking progress. This is where a solution that provides clear visibility and control becomes paramount.
The Human-Agent Interface: Bridging the Communication Gap
A critical challenge lies in effectively bridging the communication gap between human team members and AI Agents. When agents operate as black boxes, lacking transparency in their decision-making processes, developers and data scientists struggle to understand their rationale and identify potential errors. This lack of transparency can erode trust in the agent's capabilities and hinder its adoption within the team. Imagine an AI Agent automatically assigning tasks based on perceived skill sets. If the agent's criteria are opaque, developers may feel unfairly burdened or overlooked, leading to resentment and decreased productivity.
Moreover, the absence of clear feedback mechanisms prevents AI Agents from learning and adapting to the team's specific needs and preferences. Without explicit guidance and feedback, the agent may continue to make suboptimal decisions, perpetuating inefficiencies and hindering project progress. Consider a scenario where an AI Agent is responsible for triaging bug reports. If the agent misclassifies critical bugs as low priority, the team may inadvertently delay their resolution, leading to customer dissatisfaction and potential revenue loss. The integration of AI Agents requires careful consideration of the human-agent interface to ensure seamless collaboration and maximize their potential benefits. These challenges highlight the need for a project management solution that emphasizes transparency and facilitates clear communication between team members and AI Agents.
Orchestrating Intelligent Automation: A Strategic Approach
To effectively harness the power of AI Agents and mitigate the risks of workflow gridlock, organizations need to adopt a strategic approach that emphasizes transparency, collaboration, and continuous improvement. This involves carefully selecting the appropriate AI Agents for specific tasks, providing clear guidelines and feedback mechanisms, and fostering a culture of experimentation and learning. The key is to view AI Agents not as replacements for human team members, but as valuable tools that augment their capabilities and free them from repetitive tasks.
One crucial aspect of this approach is the implementation of robust project management tools that provide clear visibility into the agent's activities and outputs. This allows developers and data scientists to easily monitor the agent's performance, identify potential errors, and provide timely feedback. Furthermore, project management tools can facilitate seamless collaboration between human team members and AI Agents, ensuring that everyone is aligned on project goals and priorities.
GitScrum, for example, offers a comprehensive suite of features that enable teams to effectively manage their projects, track progress, and collaborate seamlessly. Its task management capabilities allow teams to break down complex projects into smaller, manageable tasks, assign ownership, and track dependencies. The platform's workflow visualization features provide a clear overview of the project's progress, allowing teams to identify potential bottlenecks and address them proactively. Furthermore, GitScrum's collaboration tools facilitate seamless communication between team members, ensuring that everyone is on the same page.
Harnessing Agile Methodologies with AI-Assisted Project Management
Agile methodologies, such as Scrum and Kanban, provide a flexible and iterative framework for managing complex projects. Integrating AI Agents into an agile workflow can further enhance its effectiveness by automating repetitive tasks, improving decision-making, and providing real-time insights. For example, an AI Agent could automatically generate sprint backlogs based on project requirements, prioritize tasks based on their impact, and track progress against sprint goals. This allows the team to focus on higher-level tasks, such as design, development, and testing, while the agent handles the more mundane aspects of project management.
GitScrum is specifically designed to support agile methodologies, providing teams with the tools they need to plan sprints, track progress, and manage their backlogs effectively. Its sprint planning features allow teams to estimate task durations, assign ownership, and track progress against sprint goals. The platform's Kanban board provides a visual representation of the workflow, allowing teams to identify bottlenecks and optimize their processes. By combining the power of AI Agents with the flexibility of agile methodologies, organizations can significantly improve their project outcomes and accelerate their time to market. This synergy is further enhanced by GitScrum's robust reporting and analytics capabilities, which provide valuable insights into team performance and project progress.
Consider this workflow for optimal AI agent integration within a project managed using GitScrum:
- Project Setup in GitScrum: Define project scope, objectives, and key milestones within the platform.
- Task Decomposition: Break down the project into smaller, manageable tasks and subtasks using GitScrum's task management features.
- AI Agent Integration: Identify specific tasks that can be automated or augmented by AI Agents. For example, an agent could be used to generate initial code scaffolding, preprocess data, or triage bug reports.
- Agent Configuration: Configure the AI Agent with clear guidelines, input parameters, and performance metrics. Ensure that the agent's output is aligned with the team's coding standards, data quality requirements, and project goals.
- Workflow Integration: Integrate the AI Agent into the project workflow using GitScrum's API or integration capabilities. Ensure that the agent's activities are transparent and visible to the team.
- Monitoring and Feedback: Continuously monitor the AI Agent's performance, identify potential errors, and provide timely feedback. Use GitScrum's reporting and analytics features to track the agent's impact on project outcomes.
- Iteration and Improvement: Iterate on the AI Agent's configuration and workflow integration based on feedback and performance data. Continuously improve the agent's capabilities and adapt it to the team's evolving needs.
Data-Driven Decision Making: Empowering Teams with AI Insights
One of the most significant benefits of AI Agents is their ability to analyze vast amounts of data and provide actionable insights. By leveraging machine learning algorithms, AI Agents can identify patterns, trends, and anomalies that would be difficult or impossible for humans to detect. This data-driven approach can significantly improve decision-making, optimize resource allocation, and enhance project outcomes. For example, an AI Agent could analyze code commit patterns to identify potential code quality issues, predict project risks based on historical data, or optimize resource allocation based on team skill sets and project priorities.
GitScrum's reporting and analytics features provide teams with valuable insights into project progress, team performance, and resource utilization. By integrating AI Agents with GitScrum, organizations can further enhance their data-driven decision-making capabilities and optimize their project outcomes. For example, an AI Agent could analyze GitScrum's data to identify potential bottlenecks, predict project delays, or recommend optimal resource allocation strategies. This combination of AI Agents and robust project management tools empowers teams to make informed decisions and achieve their project goals more efficiently. The key is to ensure that the data used by the AI Agents is accurate, reliable, and relevant to the project's objectives.
In essence, effective utilization of AI agents necessitates a holistic approach, integrating these tools seamlessly within a robust project management framework like GitScrum. This ensures transparency, facilitates collaboration, and ultimately empowers teams to leverage the full potential of AI without succumbing to the pitfalls of poorly integrated automation.
The integration of AI Agents into project workflows represents a significant opportunity to enhance efficiency, improve decision-making, and accelerate time to market. However, it's crucial to adopt a strategic approach that emphasizes transparency, collaboration, and continuous improvement. By leveraging robust project management tools like GitScrum, organizations can effectively manage the complexities of AI Agent integration and unlock their full potential. Ready to transform your project management? Explore GitScrum today and discover how it can empower your team to achieve greater success with AI.